Contents
- 1 projects based on ai and ml
- 2 Opening Statement:
- 3 Comprehending AI and ML: Definition of AI and ML; History and Development of AI and ML; Key Concepts and Terminology:
- 4 The evolution and history of artificial intelligence and machine learning:
- 5 Real-world Applications of AI and ML:Examples of Projects That Utilize AI and ML; Impact of AI and ML on a variety of industries; Benefits and challenges associated with the implementation of AI and ML projects:
- 6 Successful initiatives that employ AI and ML include:
- 7 The influence of AI and ML on a variety of industries:
- 8 Advantages and obstacles associated with the execution of AI and ML initiatives:
- 9 The Significance of Data in Artificial Intelligence and Machine Learning:
- 10 Data Acquisition, Cleaning, and Preprocessing:
- 11 Methods for Data Analysis and Modeling:
- 12 Algorithms for Machine Learning:
- 13 Methods of Artificial Intelligence:
- 14 The Future of AI and ML:
- 15 Machine Learning Algorithms: Popular Algorithms and Their Applications Types of machine learning algorithms: supervised, unsupervised, and reinforcement learning:
- 16 Machine learning algorithms are classified into the following categories:
- 17 1. Learning under supervision:
- 18 2. Unsupervised learning:
- 19 3. Reinforcement Learning:
- 20 Popular Algorithms and Their Applications:
- 21 Natural Language Processing (NLP), Artificial Intelligence Techniques, Computer Vision, Robotics and Automation, and Deep Learning:
- 22 An Overview of Natural Language Processing (NLP) and its Applications:
- 23 Comprehending the Application of Computer Vision in Artificial Intelligence and Machine Learning Projects:
- 24 An In-Depth Examination of the Potent AI Technique of Deep Learning:
- 25 Ethical Considerations in AI and ML Projects: Potential Ethical Issues in AI and ML Responsible and ethical development of AI and ML projects; addressing bias; and ensuring a level playing field in AI and ML systems:
- 26 The Responsible and Ethical Development of AI and ML Projects:
- 27 Ensuring Fairness and Addressing Bias in AI and ML Systems:
- 28 Advancements and current trends in artificial intelligence and machine learning:
- 29 Potential influence on the workforce and society:
- 30 Conclusion: A recap of key points Final thoughts and recommendations for further reading; encouragement for readers to explore and contribute to the world of AI and ML:
- 31 What projects can I make with AI?
- 32 Can ML and AI be used together?
- 33 What is the AI ML project?
projects based on ai and ml
Opening Statement:
I am pleased to welcome you to my site, which is dedicated to initiatives that are founded on artificial intelligence (AI). Artificial intelligence (AI) and machine learning (ML) are among the most thrilling and swiftly evolving disciplines in the contemporary world, as technology is advancing at an unprecedented rate. These state-of-the-art technologies have the capacity to transform the manner in which we interact with the world, work, and exist.
This blog will delve into the captivating realm of AI and ML and the innovative ways in which they are being employed to address real-world issues. We will explore the history, essential concepts, and terminology of AI and ML, as well as their influence on a variety of industries.
Additionally, we will examine the critical role of data in AI and ML initiatives and deliberate on the advantages and obstacles associated with their implementation.
However, what are the precise definitions of AI and ML? Artificial intelligence (AI) is the term used to describe the emulation of human intelligence in machines, which enables them to perform tasks that typically necessitate human intelligence, such as decision-making and task solving.
We will commence by comprehending the fundamentals of AI and ML, which encompass their definitions, history, and development.
Following this, we will delve into the practical implementations of AI and ML and examine several thrilling projects that are employing these technologies to address intricate issues. Therefore, let us explore the boundless potential of these technologies that are revolutionizing the industry!
Comprehending AI and ML: Definition of AI and ML; History and Development of AI and ML; Key Concepts and Terminology:
Artificial intelligence (AI) and machine learning (ML) are two of the most frequently discussed technologies in the contemporary era. They have been acquiring prominence and transforming a variety of industries, including finance, healthcare, transportation, and retail.
Nevertheless, what are the precise definitions of AI and ML? What is the origin of their existence? What are the primary concepts and terminology that are associated with them?
is encompasses self-correction, reasoning, and learning. Conversely, machine learning (ML) is a subset of artificial intelligence that concentrates on the creation of statistical models and algorithms that allow computer systems to learn from data without any explicit programming.
The evolution and history of artificial intelligence and machine learning:
AI’s origins can be traced back to the 1950s, when the term was first introduced by computer scientist John McCarthyIt experienced a surge in popularity during the 1990s as a result of the proliferation of large data sets and the improvement of computing capacity.
Key terms and concepts:
In order to comprehend AI and ML, it is crucial to be acquainted with certain fundamental concepts and terminology. These consist of:
Data: The basic information that is utilized to train and develop algorithms, data is the foundation of AI and ML.
Training is the process of instructing a computer system to execute a particular task by exposing it to data.
Algorithms: A collection of rules or instructions that a computer system implements to resolve an issue or accomplish a task.
Models are the result of an algorithm that is employed to make predictions or decisions.
Supervised learning is a form of machine learning in which the algorithm is trained on labeled data and subsequently learns to make predictions based on that data.
Neural networks are a type of machine learning model that is influenced by the structure and function of the human brain.
Deep learning is a subset of machine learning that employs numerous layers of neural networks to analyze and process data.
The significance of comprehending AI and ML in the context of project development:
AI and ML have emerged as indispensable instruments for project development in the current digital era. They can assist businesses in making more informed decisions, enhancing efficiency, and gaining a competitive advantage.
It is essential for project managers and developers to comprehend the functionality of these technologies in order to successfully execute AI and ML initiatives. They can leverage the potential of AI and ML to drive innovation and accomplish business objectives by possessing the appropriate knowledge and skills.
In the subsequent chapter, we will investigate the practical applications of AI and ML and examine several initiatives that are employing these technologies to address intricate issues and achieve success.
Real-world Applications of AI and ML:Examples of Projects That Utilize AI and ML; Impact of AI and ML on a variety of industries; Benefits and challenges associated with the implementation of AI and ML projects:
The manner in which we live and work has been transformed by artificial intelligence (AI) and machine learning (ML). These technologies are capable of analyzing enormous quantities of data, learning from it, and making decisions without human intervention.
Consequently, they have been extensively implemented in a variety of sectors, including finance, healthcare, transportation, and manufacturing.
This chapter will investigate the advantages and obstacles of implementing AI and ML projects, the influence of these technologies on various industries, and a few real-world examples of such projects.
Successful initiatives that employ AI and ML include:
1. Healthcare: The healthcare industry is utilizing AI and ML to enhance patient outcomes, treatment, and diagnosis.
ML algorithms are employed by IBM’s Watson for Oncology to analyze patient data and offer personalized treatment recommendations for cancer patients. This has resulted in enhanced diagnostic and therapeutic precision, which has ultimately saved lives.
2. Finance: The financial sector is being revolutionized by AI and ML, which are automating processes and detecting fraudulent activities.
PayPal and Mastercard are among the organizations that are employing artificial intelligence (AI) to identify and prevent fraud in real time, thereby saving their consumers millions of dollars.
This has the potential to enhance transportation efficacy and decrease accidents.
The influence of AI and ML on a variety of industries:
The effects of AI and ML on various industries have been substantial. These technologies have facilitated the accelerated and more precise decision-making processes of businesses, as well as the enhancement of consumer experiences, productivity, and efficiency.
For instance, AI-powered chatbots are being implemented in customer service to effectively address consumer inquiries and offer 24/7 assistance.
AI and ML have the potential to enhance patient outcomes in the healthcare sector by predicting potential health risks, assisting physicians in diagnosis and treatment, and identifying the most effective treatment plans.
These technologies have allowed companies in the finance sector to automate commonplace tasks, reduce errors, and enhance fraud detection.
Ultimately, self-driving vehicles have the potential to save time and resources by reducing incidents and improving traffic flow in the transportation sector.
Advantages and obstacles associated with the execution of AI and ML initiatives:
There are numerous advantages to implementing AI and ML initiatives; however, there are also obstacles that must be overcome. Some of the advantages include enhanced consumer experiences, cost savings, improved accuracy, and increased efficiency.
Nevertheless, there are also apprehensions regarding the ethical implications of these technologies, including privacy concerns and bias.
Additionally, the implementation of AI and ML initiatives necessitates a substantial investment of time, resources, and expertise.
In order to develop and maintain these systems, organizations must possess a robust data infrastructure and proficient data scientists.
Furthermore, there is a possibility that employees may resist the implementation of AI and ML due to their concerns that their positions may be eliminated.
In summary, AI and ML have already had a substantial effect on a variety of industries and have the potential to further transform them. Although these technologies provide numerous advantages, they also present obstacles that
The Significance of Data in Artificial Intelligence and Machine Learning:
AI and ML are predicated on data. The learning and performance enhancement of these technologies are contingent upon the utilization of immense quantities of data. The more data they possess, the more accurately they can predict and comprehend patterns.
This is the reason why companies such as Google and Facebook are so successful in the fields of AI and ML: they have access to vast quantities of user data.
The quality of data is equally important in AI and ML initiatives, in addition to its quantity. The data must be representative of the issue at hand, relevant, and accurate. Biased outcomes and inaccurate predictions may result from incomplete or biased data.
Consequently, it is imperative to maintain the integrity of the data during the collection and preparation processes.
Data Acquisition, Cleaning, and Preprocessing:
The process of data collection for AI and ML initiatives entails the collection of data from a variety of sources, including databases, sensors, and user interactions.
This data may be either structured (e.g., categories, statistics) or unstructured (e.g., text, images).
The data must be cleansed and preprocessed to eliminate any irrelevant information, errors, or noise after it has been collected.
The process of data cleansing entails the identification and correction of errors in the data, including absent values and inconsistent formatting.
Conversely, data preprocessing entails the conversion of the data into a format that is appropriate for analysis and modeling.
This may involve the conversion of text into numerical values or the scaling of data to a specific range.
Methods for Data Analysis and Modeling:
The data has been prepared and collected, and it is now available for analysis and modeling. The process of data analysis entails the utilization of statistical and computational techniques to investigate and extract insights from the data.
This can assist in the identification of patterns, trends, and relationships that can be used to inform the development of AI and ML models.
In contrast, data modeling entails the development of algorithms and models that can learn from the data and make predictions or decisions.
This is where the significance of the data becomes even more apparent. The efficacy and accuracy of the models can be significantly influenced by the quality and quantity of the data used to train them.
Algorithms for Machine Learning:
AI and ML initiatives employ a variety of machine learning algorithms. These consist of reinforcement learning, unsupervised learning, and supervised learning. Each algorithm has its own unique applications and strengths.
The model is trained on labeled data in supervised learning, where the desired output is predetermined. Reinforcement learning entails the model being trained through trial and error to accomplish a particular objective, such as playing a game.
Methods of Artificial Intelligence:
Other AI techniques are employed in initiatives in addition to machine learning. These encompass automation, robotics, computer vision, and natural language processing (NLP).
NLP enables machines to comprehend and produce human language, whereas computer vision enables machines to interpret and analyze visual data.
Robotics and automation are the processes of designing and programming devices to perform tasks autonomously, such as in logistics or manufacturing.
The use of biased data or algorithms can result in discriminatory outcomes, and the absence of transparency in AI decision-making can raise concerns about accountability.
Consequently, it is imperative that developers and organizations take into account the ethical implications of their AI and ML initiatives when designing and implementing them.
This encompasses the inclusion of diverse perspectives in the development process, the promotion of impartiality and transparency, and the mitigation of bias in data and algorithms.
The Future of AI and ML:
The field of AI and ML is in a state of perpetual evolution, and there are numerous thrilling developments that are imminent.
The current trends encompass the application of AI in cybersecurity, finance, and healthcare, as well as advancements in computer vision and natural language processing.
We can anticipate the emergence of even more advanced AI and ML systems in the future, which could potentially result in substantial changes to our society and workforce.
In summary,
In summary, data is essential for AI and ML initiatives. It is the propellant that propels these technologies and enables them to make precise predictions and decisions.
Data analysis and modeling are critical components of the success of these initiatives, while data acquisition, cleansing, and preprocessing are essential steps in the process.
It is imperative to take into account the ethical implications and ensure the responsible and ethical development of AI and ML as they continue to advance.
We have only begun to explore the potential of AI and ML, and the future is brimming with limitless opportunities.
Machine Learning Algorithms: Popular Algorithms and Their Applications Types of machine learning algorithms: supervised, unsupervised, and reinforcement learning:
Machine learning (ML) is a subset of artificial intelligence (AI) that concentrates on the development of algorithms and models that can learn from data and make predictions without being explicitly programmed.
It is a rapidly expanding discipline that has a diverse array of applications in a variety of sectors, including finance, healthcare, and transportation.
This chapter will investigate the various varieties of machine learning algorithms and their practical applications in real-world projects.
Machine learning algorithms are classified into the following categories:
There are three primary categories of machine learning algorithms: reinforcement learning, unsupervised learning, and supervised learning. Each variety possesses its own distinctive attributes and applications.
1. Learning under supervision:
Supervised learning is a form of machine learning in which the algorithm is trained on a labeled dataset. This implies that the data utilized for training has already been labeled with the appropriate output.
The objective of supervised learning is to develop a mapping function that connects the input variables to the output variables.
A few of the most frequently employed algorithms in supervised learning are linear regression, decision trees, and random forests.
Applications: Supervised learning is frequently employed for classification and regression tasks, including the prediction of customer attrition, fraud detection, and stock market forecasting.
2. Unsupervised learning:
Unsupervised learning entails the training of an algorithm on an unlabeled dataset. This implies that the algorithm is responsible for independently identifying patterns and relationships within the data.
In contrast to supervised learning, the algorithm is not guided by a correct output. Popular algorithms employed in unsupervised learning include association rule learning, principal component analysis (PCA), and k-means clustering.
Applications: Unsupervised learning is frequently employed for tasks such as anomaly detection, customer segmentation, and recommendation systems.
3. Reinforcement Learning:
Reinforcement learning entails the training of an algorithm to make decisions based on a reward system. The algorithm acquires knowledge through trial and error, obtaining either positive or negative feedback in accordance with its actions.
Q-learning and deep Q-networks are among the most frequently employed algorithms in reinforcement learning.
Applications: Reinforcement learning is frequently employed in autonomous systems, robotics, and game play.
Popular Algorithms and Their Applications:
1. Linear Regression:
Linear regression is a supervised learning algorithm that is employed to forecast continuous numerical values.
It operates by minimizing the distance between the data points and a line that is fitted to them. It is frequently employed in the prediction of stock prices, housing prices, and sales forecasting.
2. K-Means Clustering:
K-means clustering is an unsupervised learning algorithm that is employed to organize data elements into clusters based on their similarities.
It operates by determining the center of each cluster and subsequently designating data points to the closest center. It is frequently employed in the areas of image segmentation, text clustering, and customer segmentation.
3. Deep learning:
Using artificial neural networks to learn from data, deep learning is a subset of machine learning. It is frequently employed for duties such as autonomous driving, natural language processing, and image and speech recognition.
4. Q-Learning:
Q-learning is a reinforcement learning algorithm that is employed to make decisions in a dynamic environment. In order to optimize the reward, it must discover the most advantageous course of action in a specific state. It is frequently employed in the fields of robotics, self-driving vehicles, and game-playing.
We can anticipate the emergence of even more innovative and impactful applications of machine learning algorithms in a variety of industries as technology continues to evolve.
Natural Language Processing (NLP), Artificial Intelligence Techniques, Computer Vision, Robotics and Automation, and Deep Learning:
Artificial intelligence (AI) and machine learning (ML) have become indispensable components of our daily existence in the current digital era.
AI and ML are transforming the way we interact with technology, from self-driving vehicles to virtual assistants such as Siri and Alexa.
The advancement of advanced AI techniques, including deep learning, computer vision, robotics and automation, and natural language processing (NLP), is one of the primary factors driving this transformation.
An Overview of Natural Language Processing (NLP) and its Applications:
9NLP is a subfield of AI that concentrates on the ability of machines to comprehend and interpret human language.
It entails instructing computers to analyze, interpret, and generate human language in a manner that is indistinguishable from the manner in which humans communicate.
There is an extensive array of applications for NLP, such as sentiment analysis, language translation, chatbots, and voice recognition.
With the proliferation of chatbots and virtual assistants, natural language processing (NLP) has become an indispensable instrument for businesses to optimize efficiency and enhance the customer experience.
Comprehending the Application of Computer Vision in Artificial Intelligence and Machine Learning Projects:
Another essential AI technique is computer vision, which allows machines to comprehend and interpret visual information from images or videos.
It entails instructing computers to identify and categorize objects, individuals, and actions in images and recordings.
This technology has a wide range of practical applications, including medical imaging, facial recognition, and self-driving vehicles.
Computer vision has become an essential component of a variety of AI and ML initiatives as a result of the improvements in AI and ML. In particular, it has become more precise and efficient.
The Revolutionization of the Field of Robotics and Automation: The Role of AI and ML
The discipline of robotics and automation is also being transformed by AI and ML. Robots are capable of performing intricate duties with precision and adapting to evolving environments with the assistance of these new technologies.
This has resulted in the creation of sophisticated machines that can collaborate with humans in sectors including logistics, healthcare, and manufacturing.
AI and ML have also enhanced the intelligence of automation by allowing machines to learn and enhance their processes, resulting in increased productivity and efficiency.
An In-Depth Examination of the Potent AI Technique of Deep Learning:
Deep learning is a subset of machine learning that entails the training of artificial neural networks to learn and make decisions independently.
In recent years, this technique has garnered substantial attention for its capacity to generate precise predictions and analyze vast quantities of data.
Deep learning has been effectively implemented in a variety of domains, such as autonomous vehicles, natural language processing, and speech and image recognition.
Deep learning’s potential is boundless as computing power and data availability continue to improve.
In summary, the integration of these sophisticated AI methodologies has enabled the development of innovative projects across a variety of sectors.
AI and ML have the capacity to revolutionize healthcare and enhance the consumer experience, thereby transforming our world in ways that were previously unimaginable.
Nevertheless, it is imperative to take ethical considerations into account when developing and implementing AI and ML projects, as with great power comes great responsibility.
Ethical Considerations in Artificial Intelligence and Machine Learning Projects:
It is imperative to confront potential ethical dilemmas that may arise as AI and ML continue to develop.These include apprehensions regarding the workforce, bias, and privacy.
It is imperative that organizations and developers prioritize the responsible and ethical development of AI and ML projects. This encompasses the promotion of transparency regarding the data utilized and the guarantee of impartiality in the decision-making process.
The Future of AI and ML:
The future of AI and ML is brimming with potential. In the years ahead, we can anticipate the emergence of even more innovative projects and applications due to the ongoing advancements in technology.
Nevertheless, it is also crucial to take into account the potential impact on the workforce and society. It is imperative to anticipate potential changes in the employment market as machines become increasingly intelligent.
In summary, AI and ML have the capacity to revolutionize our world in ways that were previously unimaginable.
There are an infinite number of opportunities for innovation and development in this discipline, and it is a thrilling time to be involved.
It is imperative to prioritize responsible development and take into account the potential impact on society as we continue to explore and push the boundaries of AI and ML.
Let us adopt this technology and apply it to the development of a more favorable future for all.
Ethical Considerations in AI and ML Projects: Potential Ethical Issues in AI and ML Responsible and ethical development of AI and ML projects; addressing bias; and ensuring a level playing field in AI and ML systems:
The impact of artificial intelligence (AI) and machine learning (ML) technologies on various industries and aspects of our daily lives is becoming increasingly apparent as they continue to mature.
AI and ML have the potential to transform the way we live and work, from enhancing efficiency and productivity to offering personalized experiences.
Nevertheless, this authority entails a significant degree of accountability, and it is imperative that AI and ML initiatives be developed and executed in a responsible and ethical manner.
This chapter will investigate the potential ethical concerns associated with AI and ML, the significance of responsible development, and the methods for addressing bias and ensuring impartiality in AI and ML systems.
Potential Ethical Concerns in AI and ML: The potential for bias and discrimination is one of the primary concerns associated with AI and ML.
AI and ML systems are only as impartial as the data they are trained on, and if the data is prejudiced, the system will generate biased results.
This can result in severe repercussions, including the misidentification of individuals in security or law enforcement environments.
The absence of transparency and explainability in AI and ML systems is an additional ethical concern. It can be difficult to comprehend the decision-making process of these technologies as they become more intricate.
This absence of transparency can result in mistrust and uncertainty, particularly in critical decision-making processes like finance or healthcare.
Privacy is also a substantial ethical concern in AI and ML initiatives. The potential for sensitive information to be exposed or misused exists due to the extensive collection and utilization of data.
This can result in severe repercussions for individuals, including discrimination based on personal information or identity fraud.
The Responsible and Ethical Development of AI and ML Projects:
It is imperative that AI and ML initiatives be developed in a responsible and ethical manner in order to address these ethical concerns.
This entails taking into account the potential impact on society and ensuring that the technology is utilized for the betterment of all.
It also entails the promotion of transparency and accountability in the development and implementation of AI and ML systems.
One approach to guaranteeing responsible development is to involve a variety of teams in the development of AI and ML initiatives.
This can assist in the identification and mitigation of potential biases in the algorithms and data that are employed. Additionally, it encourages diversity and inclusion in the development of these technologies, which may result in more equitable and ethical outcomes.
Regular testing and monitoring of AI and ML systems is another essential component of responsible development.
This can assist in the identification and mitigation of any biases that may develop during the system’s operation. It also enables the technology to be continuously improved and refined to guarantee ethical and equitable results.
Ensuring Fairness and Addressing Bias in AI and ML Systems:
There are numerous measures that can be implemented to mitigate bias and guarantee impartiality in AI and ML systems.
One method is to employ datasets that are inclusive and diverse in order to train the systems. This entails the incorporation of a diverse array of data from various demographics in order to prevent bias and discrimination.
An alternative method is to incorporate impartiality metrics into the development and testing of AI and ML systems.
These metrics can assist in the identification of any potential biases and the verification that the system is generating results that are both fair and impartial.
Additionally, bias can be mitigated and equity can be guaranteed through consistent audits and evaluations of AI and ML systems.
By fostering fairness and resolving biases in AI and ML systems, we can maximize the potential of these technologies while simultaneously reducing their negative impact on society.
It is the duty of developers, researchers, and policymakers to collaborate in order to establish a future in which AI and ML are employed ethically and responsibly for the benefit of all.
AI and ML are revolutionizing the way we live and work, from self-driving vehicles to virtual assistants.
It is crucial to comprehend the current trends and predictions for the future of AI and ML, as well as their potential impact on society and the workforce, as we continue to observe advancements in these fields.
Advancements and current trends in artificial intelligence and machine learning:
The increasing prevalence of deep learning, a subset of machine learning that employs artificial neural networks to analyze vast quantities of data and derive insights, is one of the most significant trends in artificial intelligence and machine learning.
This has resulted in significant advancements in robotics, natural language processing, and speech and image recognition.
An additional trend is the integration of AI and ML into a variety of sectors, including finance, healthcare, transportation, and retail.
This has resulted in the creation of new employment opportunities and an increase in efficiency and productivity.
This could have significant implications for industries such as healthcare, where AI and ML could aid in diagnosis and treatment, potentially sparing innumerable lives.
Potential influence on the workforce and society:
The potential impact on society and the workforce has prompted concerns as a result of the rise of A and ML.
Although these technologies have the potential to enhance our lives in numerous ways, there are also apprehensions regarding the expansion of the digital divide and job displacement.
It is crucial for governments and organizations to resolve these concerns and guarantee that the advantages are available to all as AI and ML become more prevalent in a variety of industries.
It is also essential to think about the ethical implications of AI and ML. Biased decision-making and the reinforcement of societal inequalities are potential consequences of the increasing sophistication of these technologies.
It is crucial for developers and organizations to prioritize the responsible and ethical development of AI and ML systems, as well as to recognize and mitigate any potential biases and guarantee the impartiality of their algorithms.
In summary,
In summary, the future of AI and ML is both thrilling and uncertain. It is crucial to address potential ethical concerns and guarantee that the advantages are accessible to all, despite the fact that we can anticipate further advancements and the integration of these technologies into a variety of industries.
It is imperative that individuals and organizations remain informed and contribute to the responsible and ethical development of AI and ML as we progress.
AI and ML have the potential to significantly benefit society and the workforce when implemented correctly. It is important to acknowledge their potential while also considering their potential impact.
Conclusion: A recap of key points Final thoughts and recommendations for further reading; encouragement for readers to explore and contribute to the world of AI and ML:
In this blog, we have investigated the captivating realm of AI and ML and the ways in which they are revolutionizing industries and our daily lives.
The definition and history of AI and ML, as well as important concepts and terminology, have been the subject of our discussion.
AI is the simulation of human intelligence processes by machines, and ML is a subset of AI that allows systems to learn and develop from experience without being explicitly programmed. Initially, we defined AI as these concepts.
Additionally, we acquired knowledge regarding the history and evolution of AI and ML, which spans from the earliest rule-based systems to the most recent developments in deep learning and neural networks.
Subsequently, we investigated real-world applications of AI and ML, which included initiatives in a variety of sectors, including finance, healthcare, and transportation.
Additionally, we deliberated on the potential employment displacement and enhanced efficiency and productivity that AI and ML could have in these sectors.
Subsequently, we explored the significance of data in AI and ML projects, underscoring the necessity of high-quality data to achieve precise and effective outcomes.
Additionally, we acquired knowledge regarding data capture, cleansing, and preprocessing techniques, as well as model-building and analysis methodologies.
Next, we deliberated on the various varieties of machine learning algorithms, such as reinforcement learning, unsupervised learning, and supervised learning, and their respective applications.
Additionally, we investigated prevalent algorithms, including neural networks, support vector machines, and decision trees.
We subsequently examined a variety of artificial intelligence techniques, such as computer vision, robotics, automation, and natural language processing (NLP).
These methods are employed to facilitate the comprehension and interaction of machines with their surroundings, resulting in progress in fields such as autonomous vehicles, speech recognition, and image recognition.
In addition, we addressed the ethical considerations in AI and ML initiatives, emphasizing potential issues such as bias and the responsible and ethical development of these technologies.
Additionally, we deliberated on the significance of guaranteeing transparency and equity in artificial intelligence (AI) and machine learning (ML) systems to prevent adverse outcomes.
Lastly, we examined the future of AI and ML, examining current trends and advancements as well as making predictions for the future.
Additionally, we discussed the potential influence of these technologies on society and the workforce, underscoring the necessity of ongoing learning and adaptation to remain current with the swiftly changing landscape of AI and ML.
In summary, the world in which we reside is undergoing a rapid transformation as a result of AI and ML, and the potential applications are limitless.
Here are some final thoughts and recommendations for further reading as you continue to learn and investigate the world of AI and ML:
Maintain awareness of the most recent advancements and developments in AI and ML by participating in seminars, conferences, and online resources.
Apply your knowledge by engaging in personal initiatives or contributing to open-source projects.
Remain informed about the ethical considerations and responsible development practices in AI and ML.
The demand for competent professionals in the fields of AI and ML is on the rise, so it may be worth considering a career in this field.
AI and ML are here to stay, and it is a thrilling time to be a part of this swiftly evolving discipline. In summation.
Therefore, let us persist in our exploration and contribution to the field of AI and ML and observe the direction in which this voyage leads. Main Points:
Artificial intelligence (AI) is a branch of computer science that concentrates on the development of intelligent devices that are capable of completing tasks that are typically associated with human intelligence.
Machine learning (ML) is a subset of artificial intelligence that entails the training of machines to learn from data and make predictions or decisions without being explicitly programmed.
The utilization of data is essential in AI and ML initiatives, and techniques such as data acquisition, cleansing, and preprocessing are essential for reliable results.
There are a variety of machine learning algorithms, such as supervised, unsupervised, and reinforcement learning, each with its own set of applications and advantages.
A wide range of applications are available as AI techniques, including natural language processing, computer vision, and robotics, are rapidly advancing.
Despite the significant potential of AI and ML, it is imperative to address ethical concerns and issues such as bias and impartiality in their development and use.
Nevertheless, it is imperative to approach their development and use with a responsible attitude, taking into account the ethical implications and potential repercussions.
As individuals, we can make a meaningful contribution to the field of AI and ML by remaining informed and investigating opportunities to learn and employ these technologies.
It is incumbent upon us, as professionals, to guarantee the ethical and responsible advancement of AI and ML initiatives.
Suggestions:
There are a variety of resources available to those who are interested in learning more about AI and ML, such as online courses, books, and research papers. Additionally, it is imperative to remain informed about the most recent developments and trends in the field.
Furthermore, participating in hackathons and conferences, as well as contributing to open-source initiatives, can broaden your knowledge and technical abilities.
We should persist in our efforts to investigate the extensive potential of AI and ML and apply it to the improvement of the world for all.
In summary, AI and ML have already had a substantial impact on a variety of industries and will continue to influence the future.
As we continue to develop these technologies, it is imperative that we prioritize responsible and ethical development to guarantee that their advantages are accessible to all.
I am grateful for your company as we embark on this expedition to investigate the realm of AI and ML. I trust that this blog has offered you valuable insights and has piqued your interest in acquiring additional knowledge.
We should continue to embrace and contribute to the constantly changing world of AI and ML.
What projects can I make with AI?
Artificial intelligence, or AI, is a rapidly expanding discipline that has the potential to transform the way we live and work. AI is already having a significant impact on our daily existence, from self-driving automobiles to virtual assistants.
If you are a novice, you may be uncertain about the types of projects that can be developed with AI and how to commence. We will provide a step-by-step guide on how to construct your own fundamental AI project and introduce you to some straightforward AI projects for novices in this blog.
However, it is imperative that we first comprehend the fundamentals of AI and the reasons why it is worthwhile to acquire knowledge.
What is artificial intelligence?AI is a field of computer science that concentrates on the development of intelligent machines that are capable of completing tasks that are typically associated with human intelligence.
This may encompass a variety of activities, such as problem-solving, decision-making, and learning. Machine learning is a process by which AI systems can continually enhance their performance by utilizing algorithms and data to make predictions and decisions.
AI: What is the benefit?The demand for experienced professionals is considerable in the swiftly expanding field of AI. The acquisition of AI skills can provide access to a plethora of employment opportunities in a variety of sectors, such as finance, healthcare, and technology.
Furthermore, AI has the capacity to enhance efficacy in numerous aspects of our lives and resolve intricate issues, rendering it a valuable skill to possess.
An Overview of Artificial Intelligence Programming LanguagesPython and Java are two of the most frequently employed programming languages in the development of artificial intelligence.
Python is a language that is extensively used in AI and machine learning applications and is user-friendly for beginners.
It is effortless to implement AI algorithms due to its extensive library and framework collection and straightforward syntax.
However, Java is a potent language that is employed in a diverse array of applications, such as artificial intelligence. It provides high efficacy and scalability, despite its steeper learning curve.
Advantages of Implementing Artificial Intelligence in ProjectsThe integration of AI into your initiatives can result in a variety of advantages, including enhanced efficiency, accuracy, and decision-making.
Additionally, artificial intelligence (AI) can enable you to concentrate on more critical aspects of your project by automating repetitive duties, thereby saving time and resources.
These assignments will provide you with practical experience in AI programming and assist you in acquiring a more comprehensive understanding of its concepts.
1. ChatbotA chatbot is a computer program that replicates human conversation through text or voice interactions.
Python and a natural language processing (NLP) library such as NLTK can be employed to develop a basic chatbot. The purpose of this project is to assist you in comprehending the process of processing and responding to user input, as well as the process of training your chatbot to enhance its responses.
2. Image RecognitionThe capacity of a computer to identify and classify objects in an image is known as image recognition. Python and a deep learning library such as TensorFlow or Keras can be employed to develop a fundamental image recognition project.
This assignment will instruct you on the process of training a model to identify objects in images and to generate predictions based on its training. Tutorials and installation instructions are accessible online.
Step 2: Data AcquisitionA dataset is required to train your AI model. You have the option of locating open-source datasets online or generating your own by accumulating data and annotating it appropriately.
Step 3: Data PreprocessingNext, you will need to preprocess your data by cleansing, normalizing, and formatting it in order to prepare it for training.
Step 4: Model TrainingTrain your model by supplying it with preprocessed data and altering its parameters to enhance its performance using TensorFlow.
Step 5: Evaluate the ModelAfter the model has been trained, it can be tested by introducing new data and assessing its predictions. This phase will assist you in identifying any areas in which your model could be improved.
The sixth step involves the deployment of the model.Ultimately, your model can be deployed by incorporating it into a web or mobile application, thereby enabling users to access it.
In conclusion, the field of AI is enthralling and offers an infinite number of potential applications.
As a novice, you can begin your exploration of AI by developing straightforward applications using programming languages such as Python and Java.
You can contribute to the advancement of this thrilling technology by developing more complex AI projects with practice and determination. Therefore, what AI-based initiatives will you develop? The ceiling is the limit!
Can ML and AI be used together?
Artificial intelligence is the term used to describe the emulation of human intelligence in machines that are designed to reason and behave in a manner similar to that of humans.
It entails the creation of intelligent systems that are capable of performing tasks that are typically associated with human intelligence, including speech recognition, decision-making, and problem-solving.
AI is a comprehensive discipline that includes a variety of subfields, such as robotics, natural language processing, and machine learning.
On the other hand, machine learning is a subset of AI that entails the training of machines to make predictions or decisions based on data without being explicitly programmed.
Collaboration: AI and ML are inextricably linked, and they frequently collaborate to accomplish a shared objective. The overarching framework for machines to emulate human intellect is provided by AI, while the tools and techniques to achieve this are provided by ML.
Examples of AI and ML in action in the real world:AI and ML are already having a substantial impact on a variety of industries, and their potential is boundless. AI-powered systems can analyze medical data in the healthcare sector to aid in the development of treatment plans and diagnoses.
ML algorithms are employed in the financial sector to evaluate risks and detect deception. AI is employed in the retail sector to enhance inventory management and personalize consumer experiences.
These are only a few examples of the ways in which AI and ML are enhancing the efficiency and intelligence of our world.
Key distinctions between AI and ML: Despite their frequent integration, AI and ML possess several fundamental distinctions.
Their primary distinction is their objective and methodology. ML is concerned with the training of machines to learn from data and make decisions, whereas AI is dedicated to the development of machines that are sentient and capable of human-like reasoning.
AI is a more comprehensive concept that incorporates a variety of technologies, whereas ML is a subset of AI.
Furthermore, AI is capable of functioning independently of data, whereas ML is significantly dependent on data to enhance and learn.
The significance of AI and ML in the contemporary era:It is impossible to exaggerate the significance of AI and ML in the contemporary world. These technologies have the capacity to transform industries, enhance our daily lives, and improve efficiency.
They can assist us in making more informed decisions, automating routine duties, and freeing up time for more significant work.
The potential of AI and ML is only going to increase in the future as the exponential growth of data and the advancements in computing capacity continue.
In summary, AI and ML are two potent technologies that are influencing our world and fostering innovation. However, despite their distinct distinctions, they complement one another and collaborate to enhance the efficiency and intelligence of machines.
We can anticipate the emergence of additional innovative initiatives and advancements in a variety of industries as a result of the ongoing development and adoption of AI and ML.
What is the AI ML project?
Artificial intelligence (AI) and machine learning (ML) are among the most frequently discussed topics in the contemporary world, as technology is evolving at an unprecedented rate. Although these two terms are frequently used interchangeably, they are not equivalent.
This chapter will cover the fundamentals of AI and ML projects, including their significance, the procedures required, and the most prevalent obstacles encountered during the course of these projects.
What is the definition of an AI/ML project?
A project that utilizes artificial intelligence and machine learning techniques to analyze and process data, make predictions, and provide insights is referred to as an AI/ML project.
AI is the term used to describe the emulation of human intelligence in machines that are designed to reason and behave in a manner similar to that of humans.
Conversely, machine learning (ML) is a subset of artificial intelligence (AI) that empowers machines to learn from data and enhance their performance over time through the application of statistical models and algorithms.
What is the significance of AI and ML projects?
In recent years, AI and ML initiatives have become increasingly significant as a result of their capacity to rapidly and accurately process and analyze vast quantities of data.
This has resulted in substantial progress in a variety of sectors, including finance, healthcare, and retail.
Additionally, AI and ML initiatives can automate repetitive tasks, reduce human error, and enhance efficiency, resulting in cost savings for businesses.
Procedures for an AI/ML assignment
1. Problem Identification: The initial stage in an AI/ML endeavor is to identify the problem or challenge that must be resolved.
This could encompass a wide range of applications, including the identification of financial transaction deception and the prediction of customer attrition.
2. Data Collection and Preparation: The subsequent phase involves the collection of pertinent data from a variety of sources and its preparation for analysis.
It entails the organization, cleansing, and formatting of the data to ensure that it is suitable for machine learning algorithms.
3. Data Exploration and Analysis: It is imperative to investigate and analyze the data in order to comprehend patterns and acquire insights after it has been prepared.
This procedure assists in the identification of the most pertinent variables and features for the machine learning model.
4. Model Construction and Training: In this stage, ML algorithms are employed to construct a model with the data and insights acquired in the preceding stages.
The data is subsequently used to train the model in order to make precise predictions or classifications.
5. Model Evaluation and Improvement: The model’s performance and accuracy are assessed following its construction and training. The model is retrained and adjusted if the results are unsatisfactory in order to enhance its performance.
6. Deployment and Monitoring: The final stage is to deploy the model into the real-world environment and monitor its performance.
Regular surveillance is essential for the identification of any issues or enhancements that are required to guarantee that the model continues to produce precise results.
Common obstacles encountered in AI and ML initiatives
1. Inadequate Data Quality: The success of an AI/ML endeavor is contingent upon the quality of the data used. Inaccurate results may result from incomplete, inaccurate, or biased data.
2. Algorithm Selection: The selection of the appropriate ML algorithm for a particular problem can be a difficult task due to the vast number of available algorithms. It necessitates a comprehensive comprehension of the data and the issue at hand.
3. Data Security and Privacy: The protection of sensitive data is essential in AI and ML initiatives. The business and its customers may suffer severe repercussions as a result of any data breach.
4. Interpretability: The complexity of AI and ML models can make it difficult to comprehend the process by which they make their predictions or decisions. This lack of interpretability can be a substantial obstacle, particularly in critical decision-making processes.
In summary, AI and machine learning initiatives have become an indispensable component of various industries and businesses, and their significance will only intensify.
Nevertheless, it is imperative to comprehend the fundamentals, adhere to the appropriate procedures, and be cognizant of the prevalent obstacles in order to guarantee the successful implementation and utilization of these projects.
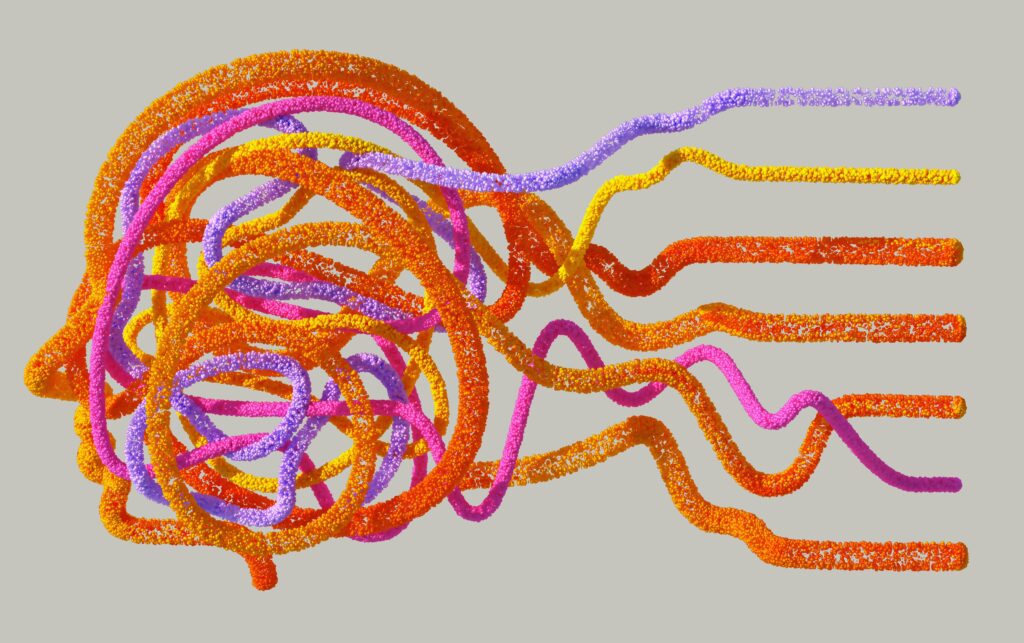